Dr. Rachel Lowe (London School of Hygiene & Tropical Medicine): modelling the impact of global e
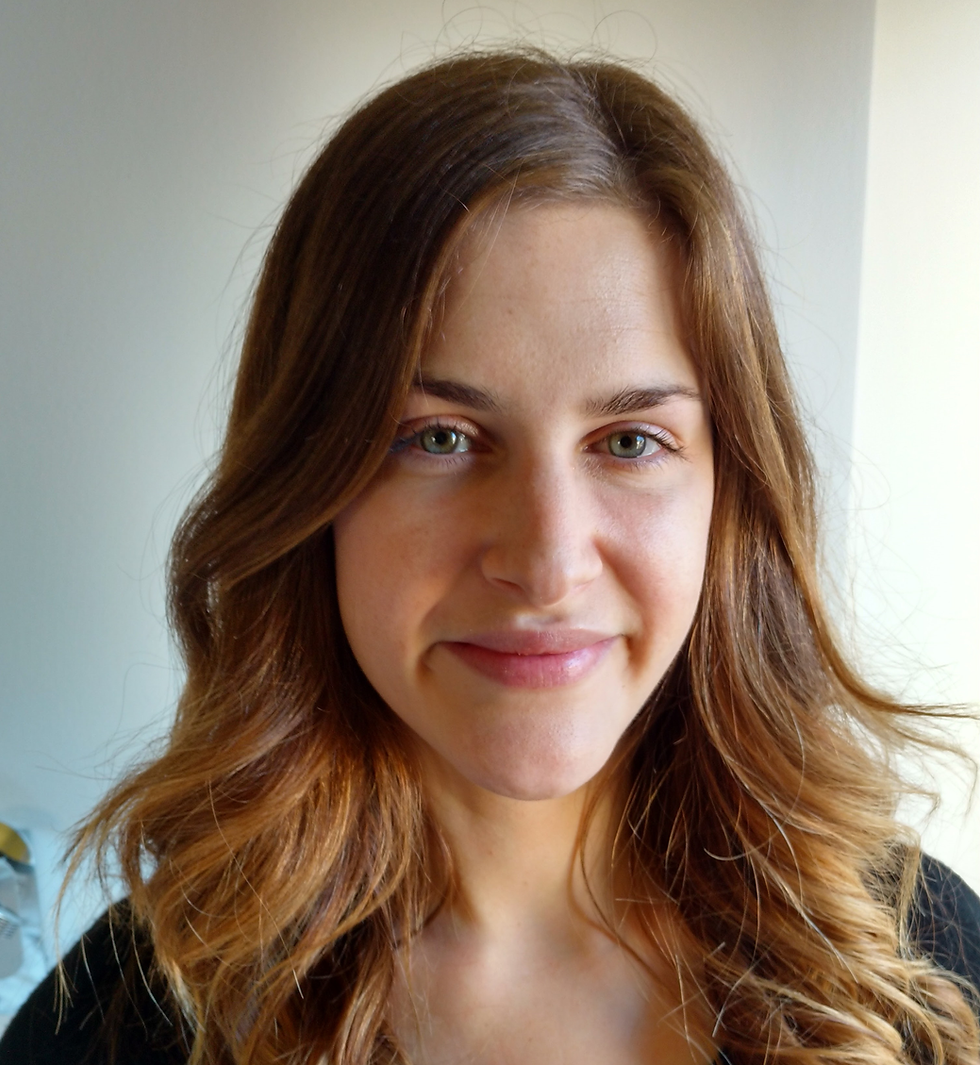
Dr. Rachel Lowe is Assistant Professor at the London School of Hygiene & Tropical Medicine and Visiting Assistant Research Professor at the Barcelona Institute for Global Health—she is currently working at the interface of climate prediction science and public health decision-making. With a doctorate in mathematics, Rachel Lowe now researches the impacts of global environmental change on vector-borne disease risk and her thesis concerned spatio-temporal modelling of climate-sensitive disease risk, with a focus on early warning systems for dengue in Brazil. In this Infectious Thoughts interview, we speak with Rachel Lowe about her thoughts on the health impacts of climatic events and what this means for neglected tropical diseases.
What are the benefits and challenges of using a Bayesian spatio-temporal analytical framework in explaining disease risk in dengue? Where do you see opportunities for this type of analysis in other neglected tropical diseases?
Statistical models based on past empirical data offer a valuable, viable, and effective approach to developing practical epidemic early warning systems. A wide range of environmental and socio-economic explanatory variables can be incorporated to understand spatio-temporal variations in disease risk, making the best use of routinely available data.
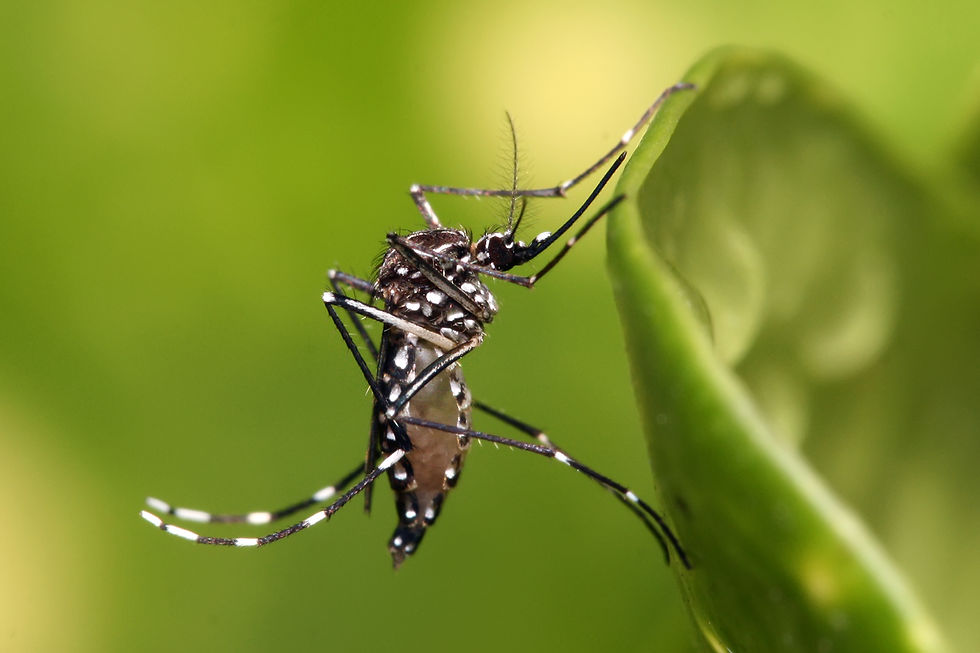
Bayesian hierarchical mixed modelling methods can help overcome potential difficulties associated with developing disease prediction models, such as accounting for unobserved confounding factors and complex spatio-temporal correlation structures. The Bayesian approach accounts for parameter uncertainty by assigning prior distributions to the parameters. Informative priors can be assigned so that previous knowledge or results can be used to inform the model. An advantage of this approach is the ability to derive full posterior predictive distributions, which automatically incorporate all components of variance at the different levels in the model.
Bayesian inference estimates a full probability model, which allows probabilistic statements about future disease risk outcomes to be derived and thorough evaluation of predictive power. A drawback of this approach is the reliance on good quality multi-source data with sufficient spatial and temporal resolution, which is not always readily available in areas with a high burden of neglected tropical diseases.
Droughts are typically slow onset (compared to floods and earthquakes) and current climatic change projections indicate that droughts will become more severe, what do you think will be the health impacts of drought and how can research inform the policies of the decision makers?
Droughts have multiple detrimental impacts of health. For example, prolong droughts can result in crop failure, which leads to food insecurity and famine. Drought increases the risk of wildfires and dust storms, which degrade air quality and exacerbate respiratory diseases. A lack of safe drinking water, sanitation and hygiene can lead to increased incidence of diarrhoeal diseases.
Research aimed at understanding the interlinked pathways and mechanisms by which drought impacts health and improving seasonal climate forecasts of drought events can help design effective early warning systems, tailored to build public health resilience to drought hazards.
What effect could extreme weather events have on the transmission of mosquito-borne diseases?
Climate change is likely to lengthen the transmission season of mosquito-transmitted diseases, such as dengue and West Nile virus. A warming climate could alter the geographical range of mosquito vectors, potentially bringing new diseases to regions which lack either population immunity or strong public health infrastructure.
More frequent extreme weather events, such as storms, floods and droughts, affect the timing and intensity of disease outbreaks. Mosquitoes thrive in warm and humid conditions with rainfall increasing the number of outdoor breeding sites. However, drought conditions can also promote breeding, due to an increase in water storage containers around the home. For example, the explosive spread of Zika virus in Brazil and the Americas in 2015-16 was attributed to a combination of factors, including a massive susceptible population along with severe drought and unusually high temperatures associated with the 2015-16 El Niño event.
What is the risk that human adaptations to the effect of water shortage (e.g water storage in containers) could have an effect on the range of Ae. aegypti vector?
Temporary water storage can have the unintended consequence of creating additional breeding sites for mosquitoes. For example, Barbados is a particularly water-scarce country, which is suffering from more frequent and severe droughts.
To address this issue, Barbados passed building regulations that mandate the construction of rainwater storage receptacles under large new buildings. However, these receptacles have become ideal larval habitat for Ae. aegypti mosquitoes and may have had the unintended consequence of increasing the overall risk of arboviral diseases, such as dengue, Zika and chikungunya.
Will reducing the effects of non-climatic factors (e.g urbanization, poor WASH practices) be sufficient enough to mitigate the impact of climate change on the distribution, burden and transmission of dengue?
Drastic improvements in infrastructure and sanitation in the poorest areas of the world are essential in combating dengue and reducing the global burden of disease. The incorporation of climate information and forecasts in public health surveillance systems can help pinpoint areas most in need of vector-control measures and build resilience against climate-related health impacts.
However, adaptation to more frequent and extreme climatic events will not be enough. Governments need to ensure a shift towards a low carbon economy to mitigate the impact of rapid climate change on human health and prevent the emergence and reemergence of infectious diseases in previously unaffected areas.